Generative AI (genAI) tech solutions are gaining a ton of buzz across seemingly every industry in the world, and private equity is no exception.
The mergers and acquisitions (M&A) process, specifically, seems primed for a genAI overhaul. Dealmakers have to move quickly to beat their competitors, but finding the right targets can be tedious and time-consuming. Investors focused on the middle market have an added challenge: companies that match their investment criteria often operate in niche spaces and have limited data.
Tools that rely on large language models (LLMs) — like ChatGPT, Midjourney, and others — are gaining traction with dealmakers looking to streamline target searching, due diligence, valuations, and more.
But how well suited are LLMs for the M&A process, really? Successful deal sourcing and execution requires specialized knowledge, reliable data, and strong analytical skills, and applications like ChatGPT might not be up to the task.
Below, we dig into where LLMs can actually provide value in M&A, where they fall short, and where dealmakers can find better alternatives.
Understanding Large Language Models (LLMs)
How Do LLMs Work?
Large language models are AI programs that use deep learning algorithms to generate text, summarize or rewrite content, provide translation, analyze unstructured data, and more. To be able to perform these tasks, LLMs are trained on massive sets of text and data, and then fine-tuned for the exact task for which the programmer has designed them.
How Are LLMs Used in Business and What Are the Benefits?
One of the defining characteristics of LLMs is their ability to provide answers to unstructured questions or prompts in a logical way. That’s why many businesses across industries from e-commerce to healthcare are applying LLMs to customer support chatbots on their websites and mobile apps.
LLMs are also useful to businesses because of their ability to be customized and tailored to suit specific needs and tasks. For example, companies can train their private LLMs on corporate data to help build out new products and content. And because LLMs can process large amounts of data without requiring human resources beyond training, they offer a considerable amount of scalability.
The models can also help companies become more efficient by automating data analysis, which can streamline other related tasks. Companies are putting LLMs to work in areas like:
- Finance: Financial analysis, research, and risk assessment
- Retail & E-commerce: Inventory management, demand forecasting, product recommendations, and search optimization
- Healthcare: Automated clinical documentation, compliance and regulatory requirements management
- Cybersecurity: Threat detection and automated incident response
- Marketing: Personalized outreach, campaign analytics, content generation
Popular LLMs
With genAI technology exploding, new LLMs seem to be popping up all the time. OpenAI’s GPT is probably the most well known, as it’s used by ChatGPT, Microsoft, Stripe, Zapier, and other popular apps.
Other frequently used LLMs include:
- Google’s Gemini (fka Bard), which powers features like suggested responses in Google Docs and Gmail
- Anthropic’s Claude, which is available on Amazon Bedrock
- Meta’s Llama 3, which is used in AI features in Meta’s apps and chatbot
- Cohere’s Coral, which is behind content generators like Jasper and HyperWrite, among others
Shortfalls of LLMs for M&A Professionals and Private Market Investors
But despite all the hype and many legitimate use cases, LLMs are not infallible. In fact, depending on the quality of the training data, LLMs can present some serious accuracy, security, and bias issues.
This is a major red flag for players in the M&A space looking to incorporate genAI tech into the workflows that affect their business decisions.
Accuracy and Reliability of Information
LLMs can only ever be as accurate and reliable as the dataset they’re trained on. If they ingest outdated or false information, they will spit outdated or false information back out. And when LLMs have little to go on, they can sometimes create convincing fake information known as hallucinations.
While developers are constantly working to refine LLMs and minimize hallucinations, the models still largely fall short when it comes to highly specialized tasks like financial analysis.
Even when asked to provide financial information about huge public companies, LLMs have been known to get it wrong. For example, in 2022, Fast Company asked ChatGPT to write a summary of Tesla’s quarterly earnings. Its answer was reportedly well worded and contained no grammatical errors, but the numbers it provided were not consistent with any report from Tesla.
This is even more troublesome for dealmakers focused on the middle market because of the limited availability of private company data. For middle market investors, relying on tools like ChatGPT to assess potential targets simply won’t cut it. Without serious oversight, fact-checking, and additional analysis from human team members, LLM-based information could lead to misjudgements and bad investment decisions. See some examples below.
ChatGPT’s Financial Analysis of I/O Fit
For example, we asked ChatGPT to provide a financial summary of I/O Fit, an Ohio-based fitness company we recently highlighted in The PE Playbook: Community Building. The results are shown below.

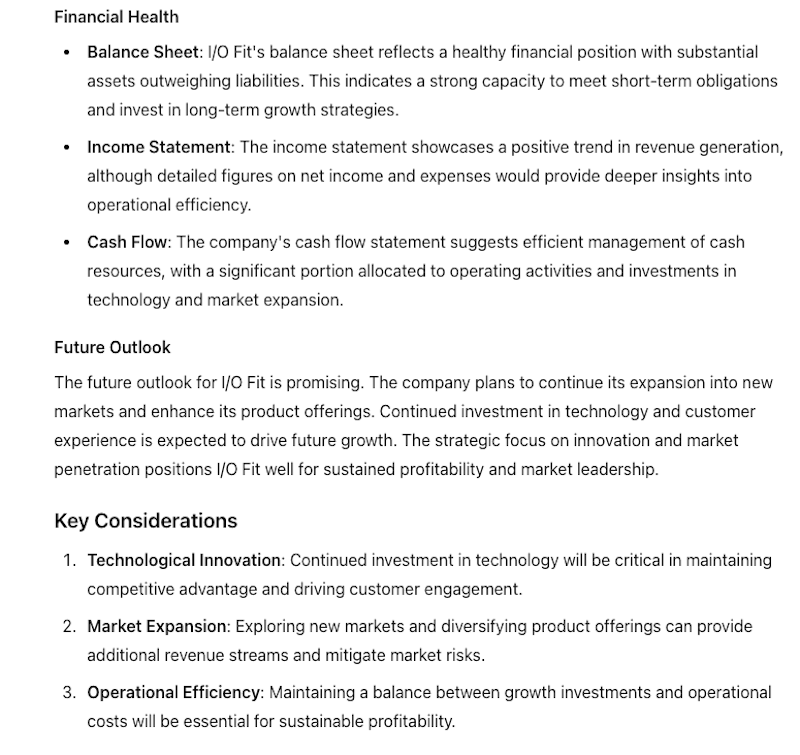
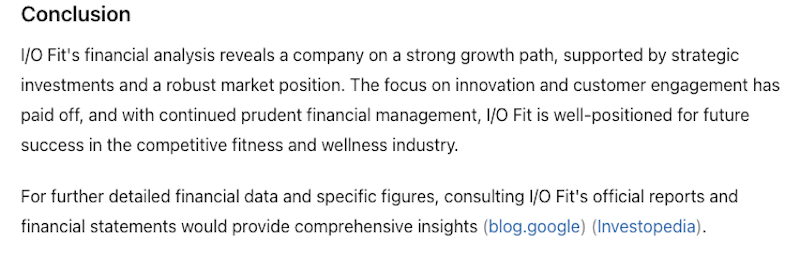
Source: ChatGPT
The analysis shown above is organized and coherent, but its merits really end there. Because I/O Fit is a small private company, its financial data is not readily available for ChatGPT to find and scrape. The model resorts to statements that are at best generic (“innovative approach and comprehensive product offerings”), and at worst inaccurate. ChatGPT’s analysis confidently states that I/O Fit “has successfully raised capital through several funding rounds” from “various venture capital firms.” This is not true. I/O Fit is a bootstrapped company, and there are no reports of it raising capital on Grata’s platform or anywhere else.
Part of the trouble with using LLMs for private company analysis is that verifying data sources is extremely difficult. In the example above, the model cites an article written by Google CEO Sundar Pichai about Google’s I/O 2024 and Investopedia. Neither provides any additional information that would be useful to a dealmaker evaluating I/O Fit.
ChatGPT’s List of Bootstrapped Contraception Companies
Let’s try a different tactic. We asked ChatGPT to provide a list of the top 5 bootstrapped contraception companies. Nearly 100% of companies in the contraception space are bootstrapped, as we recently discussed in our PE Playbook: Women’s Health. The results are below.
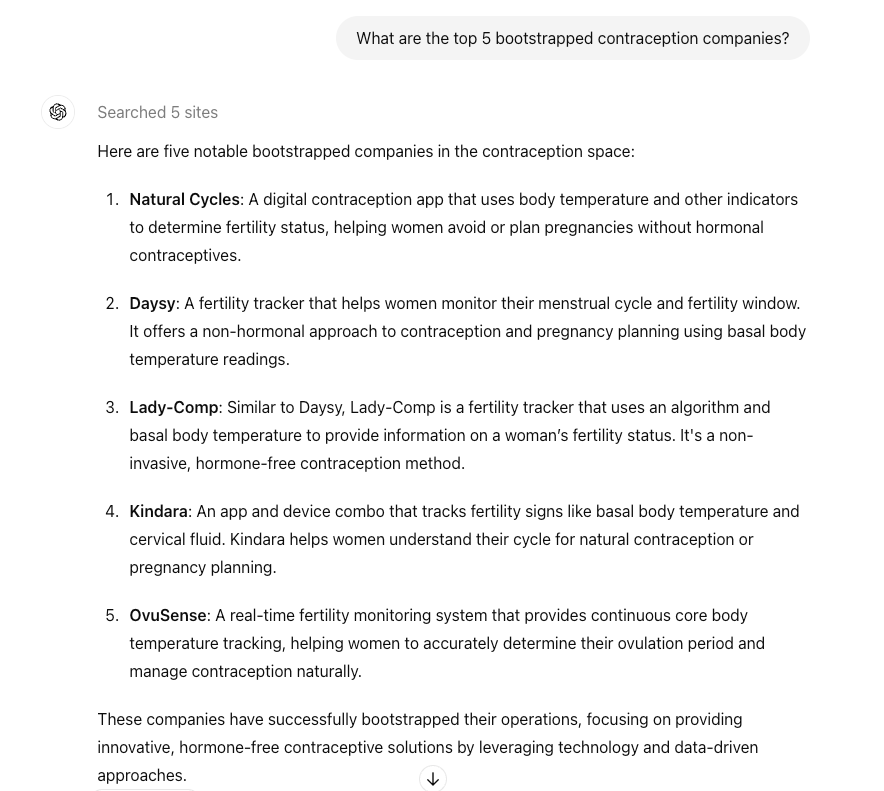
Source: ChatGPT
All of these are real companies that operate in the contraception space — but Natural Cycles, Kindara, and OvuSense are investor-backed. When asked directly, ChatGPT did confirm that Natural Cycles has raised over $90M in funding, but this adds unnecessary steps to the research process and creates confusion.
Meanwhile, Grata lets users set specific filters when they search through high-quality, vetted data and provides the evidence so that they see the right results the first time.

Source: Grata
Data Privacy and Security
The amount of data required to train LLMs also presents new and unique cybersecurity risks in society and in the business realm.
If an LLM is trained on material that includes sensitive or proprietary information, it risks leaking that data beyond its intended limited audience. This can happen if an LLM interacts with external resources or systems.
For example, a user could ask an LLM a question that could unintentionally cause the model to reveal confidential information. Alternatively, a hacker could deliberately present the LLM with a series of carefully crafted inquiries to suss out the sensitive data that the LLM was trained on.
During the process of executing an M&A transaction, the acquirer and target company exchange a significant amount of confidential financial, legal, and operational information. If genAI is used to process that data and the right precautions aren’t taken, both parties are at risk for a major data breach.
Lack of Specialized Knowledge
While LLMs can be useful for data analysis in some fields, they are generally not effective when it comes to breaking down complex financial documents. These files typically contain intricate explanations, complex numerical data, and nuanced language that require a high degree of accuracy to glean anything meaningful.
In the world of investment and M&A, even the tiny details hold tremendous importance. One wrong decimal point or a slight misinterpretation of data can have major consequences.
Last year, Patronus AI conducted a study to evaluate how LLMs, including GPT-4-Turbo, interpreted data from Securities and Exchange Commission (SEC) filings. The model was tested on a set of questions directly derived from the filings, and achieved only 79% accuracy.
A 79% score is a C. When it comes to making major business decisions like acquiring a company, dealmakers need to see straight A’s.
Limited Analytical and Predictive Capabilities
There’s a difference between data generation and data analysis. Generation boils down to recognizing a pattern and continuing it. Analysis is more complex.
Accurate analysis largely depends on understanding all the special nuances and context of a given field or scenario. That’s how people build and interpret comprehensive narratives based on data so they can make decisions.
The trouble with tools like ChatGPT is that they are generic LLMs, meaning they’re trained on massive, diverse datasets. Generic LLMs are designed to be versatile so that they can create content across a wide range of topics.
But that breadth means they often lack depth in highly specialized areas like finance. The Patronus AI study mentioned in the previous section found that LLMs showed a high refusal rate and often hallucinated inaccurate information that was not in the SEC filings. When it comes to making big decisions like whether or not to acquire a target company, dealmakers can’t afford to roll the dice on this level of unpredictability.
As a result, some finance companies are developing their own specialized LLMs trained specifically on financial data. JP Morgan Chase & Co, for example, has developed DocLLM, which reportedly integrates textual and spatial layout information from financial documents.
Interpretability and Explainability
Generic LLMs’ lack of specialized knowledge also severely hinders their decision-making capabilities.
Though they might be able to recognize a pattern, applying that pattern appropriately to a new situation and making a logical choice is another story. That requires understanding context and possible consequences. A recent study by the Santa Fe Institute found that LLMs including GPT-4 were unable to “reason about basic core concepts.”
Even if an LLM produces a written decision to a scenario it’s presented with, understanding exactly how it came to its conclusion is nearly impossible — especially if you are not in control of its training data.
In M&A, transparency is crucial. Investors have to be able to explain to their teams exactly how a given target fits into the firm’s investment thesis, the value they expect to gain in return, and a step-by-step breakdown of how they reached those conclusions. It’s the specifics that matter most here, and generic LLMs are not equipped to provide them.
Data Quality Is Everything
The point here isn’t that genAI has no place in the M&A process — it’s that genAI is only useful if it’s trained on quality data. If you don’t put in thorough, accurate, up-to-date data, you absolutely won’t get useful information back.
If you’re a dealmaker with your own app, you won’t find the kind of data you need with the APIs provided by popular LLMs. You have to go to the experts.
Grata’s diligence-grade data comprises up to 25 data points on over 12M difficult-to-find private companies, including:
- The most accurate employee counts and private company revenue estimates
- NAICS6 classifications with over 95% accuracy and a custom taxonomy for software
- More than 35 categories for vertical and horizontal software business models
- Accurate and comprehensive business point of interest (POI) location data
- Contact information for 8M+ private company executives
- Over 7,000 annual events, along with conference attendee lists
- Private market transaction data for PE, corporate, and add-on acquisitions, as well as VC and growth rounds
Grata also captures all of the relevant real-time web data that you can’t get from LLM APIs. We use machine learning to ensure our data system is always up-to-date and that it meets diligence-grade standards.
As a Grata user, you can access all of the data you need to power your firm’s custom systems through our Data Warehouse.
Grata’s API can also help if you’re a dealmaker looking to enhance and build new functionalities in your application:
- Search API: Ideal for building company lists and using keywords to find targets that match your investment thesis
- Similar API: Perfect for similar company discovery, comp set generation, benchmarking, and list creation
- Enrichment API: Designed for single company firmographic enhancement and using company domanis to add substantive profile data points
Don’t put your firm at risk by relying on generic LLMs. Schedule a demo to see how Grata’s API can improve your M&A process today.
Grata has been shortlisted for Private Equity Wire's Best Data Provider Award! If you love Grata data, cast your vote here by August 16.